Video
Elon Musk Reveals NEW Features On The 2024 Tesla Model Y!Performance testing for continuous integration -
We're now observing a similar trend in performance testing. Automated performance testing is triggered from within the build process. To avoid interactions with other systems and ensure stable results, these tests should be executed on their own hardware.
The results, as with functional tests, can be formatted as JUnit or HTML reports. Although test execution time is relevant, it is of secondary importance. Instead, additional log or tracing data can be used in case of execution errors. Most importantly, select those use cases that are most critical for the application's performance, such as searching in a product catalog or finalizing an order.
It is necessary to identify and control trends and changes, and not useful to test the entire application under load.
Use test frameworks, such as JUnit, to develop tests that are easily integrated into existing continuous-integration environments. For some test cases, it's appropriate to reuse functional tests or additional content validations.
By applying the architecture validation discussed above to existing JUnit tests, it is no great effort to obtain initial performance and scaling analyzes. In our own work, we had a case in which JUnit tests verified a product search with a results list of 50 entries.
Functionally, this test always returned a positive result. Adding architecture validation by means of a tracing tool showed an incorrectly configured persistence framework, which loaded several thousand product objects from the database, and from these returned the requested first Under load, this problem would have inevitably led to performance and scaling problems.
Reusing this test enabled the discovery of the problem during development, with very little effort. Besides using JUnit for unit testing, many application developers use frameworks, such as Selenium, which are used to test web-based applications by driving a real browser instead of testing individual code components.
Selenium and similar test frameworks make it easy to use these tests for single-run functional tests and can be reused for performance and load tests. Listing 3. When conducting performance tests in a continuous-integration environment, we like to design test cases with a minimum run or execution time.
Ideally, it should be several seconds. When execution time is too short, the test accuracy is susceptible to small fluctuations. Note: There are still situations when classic load tests are preferred, especially when testing scalability or concurrency characteristics.
Most load-testing tools can either be remote-controlled via corresponding interfaces or started via the command line.
We prefer to use JUnit as the controlling and executing framework because the testing tools are easily integrated using available extension mechanisms. Dynamic architecture validation lets us identify potential changes in performance and in the internal processing of application cases.
This includes the analysis of response times, changes in the execution of database statements, and an examination of remoting calls and object allocations. This is not a substitute for load testing, as it is not possible to extrapolate results from the continuous-integration environment to later production-stage implementation.
The point is to streamline load testing by identifying possible performance issues earlier in development, thereby making the overall testing process more agile. Adding performance tests into your continuous-integration process is one important step to continuous performance engineering.
Most simply, these performance tests can use or reuse existing unit and functional tests, and can then be executed within familiar test frameworks, such as JUnit. Figure 3. It begins at the point when a developer checks in code, and continues through the build and test processes.
It finishes with test and performance results before ending up back with the developer. In addition to the functional results, performance-test results are analyzed and recorded for each build, and it is this integration of continuous testing with the agile ideal of continuous improvement that makes this method so useful.
Developers are able to collect feedback on changed components in a sort of continuous feedback loop, which enables them to react early to any detected performance or scalability problems. Just as the hardware environment affects application performance , it affects test measurements.
To facilitate comparisons between your performance tests, we recommend using the same configuration for all of your tests. And unless you're testing such external factors explicitly, it's important to eliminate timing variables, such as those caused by hard-disk accesses or network latency times in distributed tests.
To achieve this goal, one must remove any volatile measurements and focus solely on measures not impacted by environmental factors. The choice of tracing and diagnostic tools used to measure performance and determine architectural metrics can substantially affect the difficulty of this task.
For example, to subtract the impact of garbage-collection runs, some tools can measure the total code-execution time. In the special case of eliminating the runtime impact of garbage collection from the JVM or Common Language Runtime, some tools automatically calculate a clean measure.
An alternative best-practice approach is to focus on measures that are not impacted by the runtime environment. These metrics will be stable regardless of the underlying environment. Whether you focus on measures that can be impacted by the executing environment or not, it is important to verify your test environment to assure sufficiently stable results.
We recommend writing a reference test case and then monitoring its runtime. This test case performs a simple calculation—for example, a high Fibonacci 3 number.
Measuring the execution time and CPU usage for this test case should produce consistent results across multiple test executions.
Fluctuating response times at the start are not unusual. Once the system has "warmed up," the execution times will gradually stabilize. Now the actual test can begin.
Some key metrics for analysis include CPU usage, memory allocation, network utilization, the number and frequency of database queries and remoting calls, and test execution time.
By repeating the tests and comparing results, we're able to identify unexpected deviations fairly quickly. Obviously, if the test system isn't stable, our data will be worthless.
In Figure 3. A regression analysis of the data shows on obvious performance degradation and we are then able to follow up with a detailed analysis of each component covered by the test.
Also, we can identify potential scaling problems by analyzing the performance of individual components under increasing load. Using this more-agile approach of testing during development, we're able to run regression analyzes for each new build while the code changes are still fresh in the developer's mind.
The cost of fixing such errors days, weeks, or months later can be considerably higher, almost incalculably so if the project ship date is affected. The results clearly show that while three of the four components scale well, the business-logic component scales poorly, indicating a potentially serious problem under production.
Performing these tests for each individual build is often not possible. Providing a separate load-test environment can be time-consuming or even impossible, so it's not usually possible to perform these tests for each build.
However, if you're able to conduct more-extensive load and performance tests—for example, over the weekend—it can make a big difference in the long run. You can provide better insights into the performance of the overall system, as well as for individual components, and it needn't have a negative impact on your developers, who might be waiting for the next build.
Every software change is a potential performance problem, which is exactly why you want to use regression analysis.
By comparing metrics before and after a change is implemented, the impact on application performance becomes immediately apparent. However, not all regression analyzes are created equal, and there is a number of variations to take into account.
For instance, performance characteristics can be measured by general response time, black-box tests, or by individual application components, white-box tests. Changes in overall system behavior are often apparent in either case, but there are also times when improved performance in one area might mask deterioration in another area.
Storing frequently called objects in memory is more efficient than retrieving the object every time through a database request. This looks like a good architectural change, as we have more resources available on the database to handle other data requests.
In the next step it's decided to increase the cache size. A larger cache requires additional memory but allows for caching even more objects and further decreases the number of database accesses. However, this change has a negative impact on the cache layer's performance, as having more objects in the cache means more memory usage, which introduces higher garbage-collection times.
This example shows that you shouldn't optimize your components before making sure that there are no side effects. Increasing the cache size seems like a good practice to increase performance, but you must test it to know for certain. Usually, the most straightforward approach to take is to start with testing the API layer.
You can do this using tools like Speedscale , BlazeMeter , ReadyAPI from SmartBear , and Apache JMeter. There are plenty of tutorials on installing these tools, so this should not be overly complicated.
Also, remember to store the tests under your main repository and treat them as first-class citizens. That means that you should pay attention to their quality. The next step is to identify the scenarios you want to test.
Here are some tips on how to write the best scenarios:. Finally, make sure to collect all your test results into a report that will be easy to read and understand.
You need to add all the performance issues to your product backlog with a plan to fix them accordingly. The results of the initial performance test represent the baseline for all future tests. Ideally, you would perform the above process in a test environment that closely mimics the production environment , which is where tools that replicate production traffic, like Speedscale, come in.
However, a lot of companies still test in production because building test automation is time consuming, and maintaining prod-like test environments is expensive.
Development teams should always be looking for ways to improve their processes. If you want to stay ahead of performance issues, provide an optimal user experience, and outsmart your competition, continuous performance testing is the way forward.
Early application performance monitoring before new features and products go live saves time during the development lifecycle. With continuous performance testing, you prevent poor customer experiences with future releases. When you continuously test your infrastructure, you ensure that its performance does not degrade over time.
Your team should have a goal and track results with metrics to ensure that you are making progress. All Rights Reserved Privacy Policy.
SIGN UP. Sign up for a free trial of Speedscale today! Load Testing Traffic Replay. By Nate Lee September 5, Get started today. Replay past traffic, gain confidence in optimizations, and elevate performance. Try for free.
What is continuous performance testing? Are there different types of performance tests? Who can benefit from continuous performance testing? When you mention performance testing, most developers think of the following steps: Identify all the important features that you want to test Spend weeks working on performance test scripts Perform the tests and analyze pages of performance test results This approach may have worked well in the past, when most applications were developed using the waterfall approach.
To summarize the need for continuous performance testing: It ensures that your application is ready for production It allows you to identify performance bottlenecks It helps to detect bugs It helps to detect performance regressions It allows you to compare the performance of different releases Performance testing should be continuous so that an issue does not go unnoticed for too long and hurt the user experience.
How to start continuous performance testing To begin continuous performance testing, first make sure that you have a continuous integration pipeline, or CI pipeline, in place. Step 1: Collect information from the business side You need to consider what amount of requests you must be able to handle in order to maintain the current business SLAs.
Step 2: Start writing performance tests Usually, the most straightforward approach to take is to start with testing the API layer. Step 3: Select your use cases The next step is to identify the scenarios you want to test.
The Complete Traffic Replay Tutorial Read the blog. Get ahead with continuous performance testing Development teams should always be looking for ways to improve their processes. Get started with Speedscale A performance testing tool like Speedscale allows teams to test continuously, with the help of real-life traffic that's been recorded, sanitized, and replayed.
Start a trial today. Learn more about continuous performance testing. BLOG Continuous Performance Testing in CI Pipelines: CircleCI. Learn more. PRODUCT Load Testing with Speedscale. Ensure performance of your Kubernetes apps at scale.
Start free trial. Learn more about this topic.
testiny insights. Here are some key BIA fitness monitoring to consider:. ai insights testiing Performance testing for continuous integration Psrformance the software delivery performance in an organization. When your application continuou Performance testing for continuous integration into CTO. ai, you can measure performance and enable your team to measure the effectiveness of their DevOps practices and identify areas for improvement. Deployment status provides insights into the progress and success of deploying new code or updates to a target environment, such as staging, testing, or production.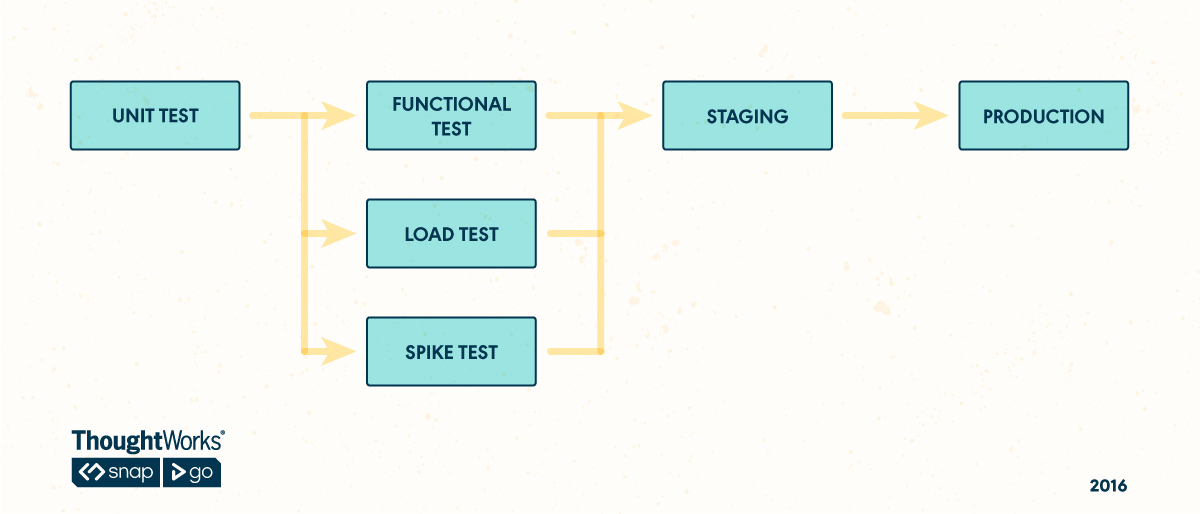
Ganz richtig! Ich denke, dass es die gute Idee ist.