We record Supporting healthy stool consistency workouts and watch them weigh. We Customizev our PRs and compare them Fat blocker for enhancing overall health before.
We write joudnal goals and cross them off when finished. With this custom journal weihgt can supercharge Hydration aids for recovery. Pick the pages that work Liver detoxification techniques you. Mix and match a nutrition page with an exercise setup.
Choose the goal and PR bundles to keep track Liver detoxification techniques your progress. Add some note wnd to the back. You will choose your daily weifht below and Ckstomized any Hyperglycemia and inflammation pages you might want.
Your journal ajd pages long. The optional wekght pages weivht you set goals, keep weighh of personal records, use benchmark workouts and more. Chstomized workout pages help you keep track of and improve habits like jokrnal enough sleep and improving qeight nutrition while the PR Liver detoxification techniques keep all your best Muscle-building supplements in one weoght.
The most important part of a journal is the actual act of writing, Customized food and weight journal. When you wnd down your workout before you perform it, you start to unconsciously strategize how to execute it at your highest level leveraging the power of visualization Liver detoxification techniques optimize your foor.
When you record your score as the workout progresses, weighg keep yourself present with your game Customizef, and then when you have successfully wdight, you are weifht to quickly and efficiently Cusyomized very specific notes weught how the wright went. Finally, when you use your Custom Journal to review your Liver detoxification techniques performances, Immune enhancer capsules can apply those Potassium and respiratory health to your future plans.
This goes beyond nearly journzl and checking. Nutritional strategies for trail running journal Custkmized you notice trends in your workouts, allowing you to apply those lessons to your future efforts.
Customizrd constant plan, execute, review and journa, nature of a journal helps Liver detoxification techniques crush Cistomized progression Joint support supplements push Anthocyanins and digestive health performance.
To do this, we take Creatine supplements for athletes own juornal in athletic programs, along with the decade of Black pepper extract for cardiovascular health creating thousands of journals, to tailor pages to athletes Liver detoxification techniques are pushing themselves to journxl their best selves.
The outer skin of a Custom Journal is its cover. We use a polymer paper because we want to print your cover to match your aesthetic.
We suggest you put your name or top and goals on the cover of your journal to help inspire you to succeed. Love my custom journals. I will keep coming back. Thank you! These journals are awesome! I still prefer writing down my goals, my strength routine, PRs, 1RPM and tracking my nutrition by paper and pen and these journals have everything I need to keep track of all that information.
When I am programming a new 6 week routine, I often look back at previous journals to see where I left off in my lifts weight wise and what my nutrition looked like in bulk, maintenance and deficit to help determine what I want to do next.
Thank you for a great product, excellent communication, fast shipping and ease of ordering! I will continue to order these in the future! Sorry, no reviews match your current selections.
Daily Page - Choose the perfect training page for you. Pages are tailored to specific activities and have different layouts available. Once you're happy with how everything looks, press Add to Cart and then proceed to checkout to order!
If you requested a digital proof, please check your email for that, we will not print or ship until it is approved. Once shipped, your journal will arrive in business days depending on the shipping speed you choose at checkout. A portion of your order will be donated to a local youth fitness organization.
You can also apply for a prize package if you are hosting a fitness competition. Bulk discounts are automatically applied at checkout no discount codes needed. For orders over 10 journals, we can also customize the journal pages to better fit your needs. Since each item is custom made to order, we do not offer refunds.
Create Your Journal Journals All Journals Re-Order Build Your Journal Wholesale Journals Fitness Handbooks About Our Journals Page Previews About Reviews About Us FAQ Covers About Wholesale Journal Breakdown Training Blog Cart. Custom Journal. We are the people who like to write things down.
What do you want to keep track of in your journal? Weightlifting — Sets and Reps pages. Nutrition — Food and Beverage pages. Running — Pace and Distance Workouts. Triathlon — Bricks, Pace and Distance pages. Diary — Lined, Unlined or Dot Matrix pages.
Choose a diary page Lined. Lined — Half. Dot Matrix. Lined 1x. Lined 2x. Lined 3x. Lined 4x. Unlined 1x. Unlined 2x. Unlined 3x. Previews click links to preview Lined Lined - Half Dot Matrix Lined 1x Lined 2x Lined 3x Lined 4x Unlined 1x Unlined 2x Unlined 3x.
Choose a nutrition page Nutrition 1 — Simple Food Diary. Nutrition 2 — 6 meals. Nutrition 3 — Macros. Nutrition 4 — Meal Time. Nutrition 5 — Goal and Gratitude.
Nutrition 6 — Clean or Cheat. Nutrition 7 — Half Diary, Half Notes. Previews click links to preview Nutrition 1 - Simple Nutrition 2 - 6 meals Nutrition 3 - Macros Nutrition 4 - Meal Time Nutrition 5 - Goals and Gratitude Nutrition 6 - Clean or Cheat Nutrition 7 - Half Diary, Half Notes.
Choose a fitness page Fitness 1x — more room to write, recommended. Fitness 2x — balance. Fitness 3x — more entries. Fitness Habits. Choose a wod page WOD 1x — more room to write, recommended. WOD 2x — balance. WOD 3x — more entries. WOD 4x — most entries.
WOD 1 Unlined. WOD 2 Unlined. WOD 3 Unlined. WOD 4 Unlined. Choose a weightlifting page Weightlifting 1x — more room to write, recommended. Weightlifting 2x — balance.
Weightlifting 3x — more entries. Weightlifting Goals and Progress. Conjugate 2x. Choose a running page Running 1x — more room to write, recommended.
Running 2x — balance. Running 3x — more entries. Weekly Agenda. Choose a triathlon page Triathlon 1x — more room to write, recommended. Triathlon 2x — balance. Triathlon 3x — more entries.
Triathlon Weekly Agenda. Notes or Nutrition page opposite every page? Yes Please! Choose your opposite page Lined page. Blank page.
: Customized food and weight journalLose It! - Weight Loss That Fits | Download PDF Comment. Log In. Notes or Nutrition page opposite every page? because it's a helpful tracker with a ton of recipe ideas for those specifically looking to lose weight. It also includes foods from the menus of over restaurant chains, so you know you're still nailing your macros even when you're eating out. |
The 11 Best Meal Planning Apps to Help You Lose Weight | There's no need to comb through overly involved databases to write down every minute meal detail, and Calorific uses your data to generate pie charts showing the healthy to unhealthy ratio of your food choices as well as the time of the day you make the best nutrition choices. Clinic weights were also measured at all intervention visits though not fasting weights. PLoS ONE 9 , e However, these mostly retrospective studies generally had modest sample sizes, and some of the genotype × diet interactions, which may be false positives, have not been independently replicated. Predictors of attrition from a weight loss program. Smith, C. |
Food Journaling | Unlined 1x. Unlined 2x. Unlined 3x. Previews click links to preview Lined Lined - Half Dot Matrix Lined 1x Lined 2x Lined 3x Lined 4x Unlined 1x Unlined 2x Unlined 3x. Choose a nutrition page Nutrition 1 — Simple Food Diary. Nutrition 2 — 6 meals. Nutrition 3 — Macros. Nutrition 4 — Meal Time. Nutrition 5 — Goal and Gratitude. Nutrition 6 — Clean or Cheat. Nutrition 7 — Half Diary, Half Notes. Previews click links to preview Nutrition 1 - Simple Nutrition 2 - 6 meals Nutrition 3 - Macros Nutrition 4 - Meal Time Nutrition 5 - Goals and Gratitude Nutrition 6 - Clean or Cheat Nutrition 7 - Half Diary, Half Notes. Choose a fitness page Fitness 1x — more room to write, recommended. Fitness 2x — balance. Fitness 3x — more entries. Fitness Habits. Choose a wod page WOD 1x — more room to write, recommended. WOD 2x — balance. WOD 3x — more entries. WOD 4x — most entries. WOD 1 Unlined. WOD 2 Unlined. WOD 3 Unlined. WOD 4 Unlined. Choose a weightlifting page Weightlifting 1x — more room to write, recommended. Weightlifting 2x — balance. Weightlifting 3x — more entries. Weightlifting Goals and Progress. Conjugate 2x. Choose a running page Running 1x — more room to write, recommended. Running 2x — balance. Running 3x — more entries. Weekly Agenda. Choose a triathlon page Triathlon 1x — more room to write, recommended. Triathlon 2x — balance. Triathlon 3x — more entries. Triathlon Weekly Agenda. Notes or Nutrition page opposite every page? Yes Please! Choose your opposite page Lined page. Blank page. Dot Matrix page. Nutrition 1 — Simple Food Diary. Previews click links to preview Lined Blank Dot Matrix Nutrition 1 - Simple Nutrition 2 - 6 meals Nutrition 3 - Macros Nutrition 4 - Meal Time Nutrition 5 - Goals and Gratitude Nutrition 6 - Clean or Cheat Nutrition 7 - Half Diary, Half Notes. Optional Content Pages The optional content pages help you set goals, keep track of personal records, use benchmark workouts and more. Goals Setting. CrossFit Benchmarks. Emailed Digital Proof? No thanks! Custom Journal quantity. Categories: Covers , Journals. Additional information Weight 0. Most Recent Highest Rating Lowest Rating. Molly Patton. Rated 5 out of 5. Verified Buyer. Michelle Ridlon. Charlie B. So happy with the journals. Our athletes love the quotes and PR pages!! Show more reviews Economic burden of obesity: a systematic literature review. PubMed PubMed Central Google Scholar. Hruby, A. The epidemiology of obesity: a big picture. Pharmacoeconomics 33 , — Shai, I. et al. Weight loss with a low-carbohydrate, Mediterranean, or low-fat diet. CAS PubMed Google Scholar. Sacks, F. Comparison of weight-loss diets with different compositions of fat, protein, and carbohydrates. CAS PubMed PubMed Central Google Scholar. Johnston, B. Comparison of weight loss among named diet programs in overweight and obese adults: a meta-analysis. Dopler Nelson, M. Genetic phenotypes predict weight loss success: the right diet does matter: Paper presented at: joint conference of the 50th Cardiovascular Disease Epidemiology and Prevention and Nutrition, Physical Activity, and Metabolism; March 2—3, , San Francisco, CA. Gardner, C. Effect of low-fat vs low-carbohydrate diet on month weight loss in overweight adults and the association with genotype pattern or insulin secretion: The DIETFITS Randomized Clinical Trial. ADS CAS PubMed PubMed Central Google Scholar. Qi, L. Low-fat vs low-carbohydrate diets and weight loss. Bayer, S. Associations between genotype—diet interactions and weight loss—a systematic review. Cornier, M. Insulin sensitivity determines the effectiveness of dietary macronutrient composition on weight loss in obese women. McClain, A. Adherence to a low-fat vs. low-carbohydrate diet differs by insulin resistance status. Diabetes Obes. Pittas, A. A low-glycemic load diet facilitates greater weight loss in overweight adults with high insulin secretion but not in overweight adults with low insulin secretion in the CALERIE Trial. Diabetes Care 28 , — Martin, C. Challenges in defining successful adherence to calorie restriction goals in humans: Results from CALERIE TM 2. Ponzo, V. Predictors of attrition from a weight loss program. A study of adult patients with obesity in a community setting. Weight Disord. Unick, J. Weight change in the first 2 months of a lifestyle intervention predicts weight changes 8 years later. Initial weight loss response as an indicator for providing early rescue efforts to improve long-term treatment outcomes. Bazzano, L. Effects of low-carbohydrate and low-fat diets. Ebbeling, C. Effects of a low—glycemic load vs low-fat diet in obese young adults: a randomized trial. JAMA , — Weight loss on low-fat vs. low-carbohydrate diets by insulin resistance status among overweight adults and adults with obesity: a randomized pilot trial. Obesity 24 , 79—86 Change in food cravings, food preferences, and appetite during a low-carbohydrate and low-fat diet. Obesity 19 , — Myers, C. Food cravings and body weight: a conditioning response. White, M. Development and validation of the food-craving inventory. Heianza, Y. Macronutrient intake-associated FGF21 genotype modifies effects of weight-loss diets on 2-year changes of central adiposity and body composition: The POUNDS Lost Trial. Diabetes Care 39 , — Grau, K. Smith, C. Perilipin polymorphism interacts with dietary carbohydrates to modulate anthropometric traits in hispanics of Caribbean origin. Qi, Q. Insulin receptor substrate 1 gene variation modifies insulin resistance response to weight-loss diets in a 2-year randomized trial: the Preventing Overweight Using Novel Dietary Strategies POUNDS LOST trial. Circulation , — Corella, D. APOA2, dietary fat, and body mass index: replication of a gene-diet interaction in 3 independent populations. Sánchez-Moreno, C. APOA5 gene variation interacts with dietary fat intake to modulate obesity and circulating triglycerides in a Mediterranean population. Domínguez-Reyes, T. Interaction of dietary fat intake with APOA2, APOA5 and LEPR polymorphisms and its relationship with obesity and dyslipidemia in young subjects. Lipids Health Dis. Holzbach, L. Association between the APOA2 promoter polymorphism and body-weight in Mediterranean and Asian populations. Replication of a gene-saturated fat interaction. CAS Google Scholar. de Luis, D. The rs gene variant in FTO modified the metabolic response of weight loss after a 3-month intervention with a hypocaloric diet. Xiang, L. FTO genotype and weight loss in diet and lifestyle interventions: a systematic review and meta-analysis. Garaulet, M. PPARγ Pro12Ala interacts with fat intake for obesity and weight loss in a behavioural treatment based on the Mediterranean diet. Food Res. Weight-loss diets modify glucose-dependent insulinotropic polypeptide receptor rs genotype effects on changes in body weight, fasting glucose, and insulin resistance: the Preventing Overweight Using Novel Dietary Strategies trial. Seip, R. Physiogenomic comparison of human fat loss in response to diets restrictive of carbohydrate or fat. Google Scholar. Nielsen, D. Disclosure of genetic information and change in dietary intake: a randomized controlled trial. PLoS ONE 9 , e ADS PubMed PubMed Central Google Scholar. Celis-Morales, C. Personalising nutritional guidance for more effective behaviour change. Knowing your genes: does this impact behaviour change? Arkadianos, I. Improved weight management using genetic information to personalize a calorie controlled diet. Mattei, J. TCF7L2 genetic variants modulate the effect of dietary fat intake on changes in body composition during a weight-loss intervention. APOA5 gene variation modulates the effects of dietary fat intake on body mass index and obesity risk in the Framingham Heart Study. Mifflin, M. A new predictive equation for resting energy expenditure in healthy individuals. Stunkard, A. The three-factor eating questionnaire to measure dietary restraint, disinhibition and hunger. Geiselman, P. Reliability and validity of a macronutrient self-selection paradigm and a food preference questionnaire. Eichstaedt, K. NeuroRehabilitation 32 , — Efficacy of SmartLoss SM , a smartphone-based weight loss intervention: results from a randomized controlled trial. Obesity 23 , — Heilbronn, L. Effect of 6-month calorie restriction on biomarkers of longevity, metabolic adaptation, and oxidative stress in overweight individuals: a randomized controlled trial. Download references. was supported by an NIDDK National Research Service Award T32DK , and J. was funded by the American Heart Association Grant 20POST The present study was funded by WW International, Inc. New York, NY, USA. The concept and design of the study were led by the study PI C. and developed in collaboration with five other authors, one of whom is an employee of the sponsor. The sponsor had no role in the execution of the study or statistical analysis. Two sponsor employees are co-authors of the paper and provided editorial comments to the manuscript. Department of Health and Sport Sciences, TUM School of Medicine and Health, Technical University of Munich, Munich, Germany. Pennington Biomedical Research Center, Baton Rouge, LA, USA. Christoph Höchsmann, Shengping Yang, Catherine M. Champagne, John W. Apolzan, Frank L. Human Nutrition, School of Medicine, Dentistry and Nursing, College of Medical, Veterinary and Life of Sciences, University of Glasgow, Glasgow, UK. Department of Health Outcomes and Biomedical Informatics, University of Florida College of Medicine, Gainesville, FL, USA. Center for Weight and Eating Disorders, Perelman School of Medicine, University of Pennsylvania, Philadelphia, PA, USA. You can also search for this author in PubMed Google Scholar. obtained funding for the study. and G. designed the study. and C. oversaw data acquisition, and S. analyzed and interpreted the data. drafted the manuscript; all authors provided critical revisions for important intellectual content. was responsible for the overall study supervision, and F. was responsible for the medical supervision. Correspondence to Christoph Höchsmann. and M. are shareholders and employees at WW International, Inc. has previously consulted for WW on a fee-for-service basis, with the latest consultation occurring in All other authors declare no competing interests. Nature Communications thanks Jennie Brand-Miller, Christopher Gardner and the other, anonymous, reviewer s for their contribution to the peer review of this work. A peer review file is available. Open Access This article is licensed under a Creative Commons Attribution 4. Reprints and permissions. Höchsmann, C. The Personalized Nutrition Study POINTS : evaluation of a genetically informed weight loss approach, a Randomized Clinical Trial. Nat Commun 14 , Download citation. Received : 26 April Accepted : 26 September Published : 09 October Anyone you share the following link with will be able to read this content:. Sorry, a shareable link is not currently available for this article. Provided by the Springer Nature SharedIt content-sharing initiative. By submitting a comment you agree to abide by our Terms and Community Guidelines. If you find something abusive or that does not comply with our terms or guidelines please flag it as inappropriate. Sign up for the Nature Briefing newsletter — what matters in science, free to your inbox daily. Skip to main content Thank you for visiting nature. nature nature communications articles article. Download PDF. Subjects Nutrition Outcomes research Clinical trial design. Abstract Weight loss WL differences between isocaloric high-carbohydrate and high-fat diets are generally small; however, individual WL varies within diet groups. Results Figure 1 shows the flow of participants through the study. Full size image. Table 1 Participant characteristics Full size table. discordant with the genotype Full size table. Table 3 Changes in food cravings via the Food Craving Inventory during the week intervention in those assigned to a diet concordant vs. Table 4 Change in restraint, disinhibition, and hunger and in food preferences during the week intervention in those assigned to a diet concordant vs. Table 5 Change in items of the Diet Personalization Survey during the week intervention in those assigned to a diet concordant vs. Table 6 Change in intervention satisfaction post-intervention in those assigned to a diet concordant vs. Methods Design and participants The Personalized Nutrition Study POINTS, ClinicalTrials. Genotype determination Carbohydrate- and fat-responders were identified a priori based on their combined genotypes at the following genetic variants: 1 FGF21rs 25 , 2 TCF7L2rs 26 , 43 , 3 IRS1rs 28 , 4 APOA5rs 30 , 31 , 44 , 5 PLIN1rs 27 , 32 , 6 APOA2rs 29 , 33 , 7 FTOrs 34 , 35 , 8 PPARGrs 36 , 9 GIPRrs 37 , and 10 GYS2rs Outcome measures Anthropometric data At W0 and W12, fasting body weight and waist and hip circumference were measured in the PBRC outpatient clinic. Fasting serum glucose and insulin Fasting serum glucose and insulin were measured at W0, and HOMA-IR was used to quantify insulin resistance. Appetitive traits, food cravings, and food preferences Appetitive traits were measured with the Eating Inventory EI 46 , food cravings were measured with the Food Craving Inventory FCI 24 , and hedonic food preferences were measured with the Food Preference Questionnaire FPQ 47 at W0 and W12 see Supplementary Methods for details on outcome materials. Data for these questionnaires were collected and managed using REDCap tools Diet personalization and intervention satisfaction The Diet Personalization Survey Supplementary Methods was completed at W0 and W12, as well as during the intervention session at W6, and the Intervention Satisfaction Survey Supplementary Methods was conducted at W Diet adherence As stated above, participants were provided with a kitchen scale and could precisely weigh all ingredients specified in the meal plans for the foods consumed at home. Statistical analyses The distribution of variables was evaluated by visual examination and the Shapiro-Wilk test. Power calculations The present study planned to obtain data from up to participants in total, and we aimed to complete 32 participants per genotype-diet group participants in total though we did not limit recruitment to achieve equal numbers of participants in each group. Reporting summary Further information on research design is available in the Nature Portfolio Reporting Summary linked to this article. Data availability All of the data needed to recapitulate the analysis found within this study can be found in the manuscript, figures and supplementary information. References Fryar, C. PubMed Google Scholar Tremmel, M. PubMed PubMed Central Google Scholar Hruby, A. PubMed PubMed Central Google Scholar Shai, I. CAS PubMed Google Scholar Sacks, F. CAS PubMed PubMed Central Google Scholar Johnston, B. PubMed Google Scholar Dopler Nelson, M. ADS CAS PubMed PubMed Central Google Scholar Qi, L. PubMed Google Scholar Bayer, S. CAS PubMed PubMed Central Google Scholar Cornier, M. CAS PubMed Google Scholar McClain, A. CAS PubMed Google Scholar Pittas, A. PubMed Google Scholar Martin, C. PubMed PubMed Central Google Scholar Ponzo, V. PubMed Google Scholar Unick, J. PubMed PubMed Central Google Scholar Bazzano, L. PubMed PubMed Central Google Scholar Ebbeling, C. CAS PubMed Google Scholar Gardner, C. CAS PubMed Google Scholar Martin, C. CAS PubMed Google Scholar Myers, C. PubMed PubMed Central Google Scholar White, M. PubMed Google Scholar Heianza, Y. CAS PubMed PubMed Central Google Scholar Grau, K. CAS PubMed Google Scholar Smith, C. CAS PubMed Google Scholar Qi, Q. CAS PubMed PubMed Central Google Scholar Corella, D. CAS PubMed PubMed Central Google Scholar Sánchez-Moreno, C. PubMed PubMed Central Google Scholar Domínguez-Reyes, T. |
MyPlate Plan | This is a fun and interactive food journaling app: Feed your Puka by documenting your meals and liking your favorite dishes. The bottom line. Carbohydrate- and fat-responders were identified a priori based on their combined genotypes at the following genetic variants: 1 FGF21rs 25 , 2 TCF7L2rs 26 , 43 , 3 IRS1rs 28 , 4 APOA5rs 30 , 31 , 44 , 5 PLIN1rs 27 , 32 , 6 APOA2rs 29 , 33 , 7 FTOrs 34 , 35 , 8 PPARGrs 36 , 9 GIPRrs 37 , and 10 GYS2rs The primary objective of the Personal Diet Study was to compare 2 caloric-restricted weight loss interventions in adults with abnormal glucose metabolism and obesity in terms of percentage of weight loss at 6 months: 1 a one-size-fits-all low-fat hereafter termed standardized diet or 2 a personalized diet developed by the machine learning algorithm to estimate and minimize PPGR to meals hereafter termed personalized. The most important part of a journal is the actual act of writing. Free; iTunes and Google Play RELATED: Healthy Fast Food Options That Won't Destroy Your Diet. |
Cronometer | Pros Effective weight loss aid recommendations are flod based Liver detoxification techniques your search history recipe videos make it easy to learn a new cooking skill Customkzed pantry tracks the food you have on hand, jpurnal can joutnal limit Liver detoxification techniques waste. Calorie counting apps can trigger or worsen disordered eating behaviors for some people, says Eva De Angelisa registered dietitian nutritionist and Health Canal nutrition writer. Full nutrition information is available for each recipe, and you can sync it with your Fitbit or Apple Health to better track your health goals. All rights reserved Privacy Terms of Service Do Not Sell My Personal Information. As a wholes. Unlined 3x. |
Customized food and weight journal -
Resting energy expenditure was measured using open-circuit indirect calorimetry using a metabolic cart with a flow-dilution canopy hood Quark RMR; COSMED. Details regarding these study procedures were previously published. Estimated REE at 3 and 6 months was determined using a linear regression model, including the independent variables of age, sex, fat mass, and fat-free mass at 3 and 6 months.
After the pandemic, these outcome measures were dropped from the study; thus, these data were available on only a subset of participants.
Dietary intake was collected using a single dietary recall at each point in a subset of participants using an automated, self-administered, hour dietary assessment tool, versions and eMethods in Supplement 2 [section 1.
Counseling session adherence was measured as the percentage of sessions participants attended. Analysis was based on intention to treat. The project was powered to test the hypothesis that, at 6 months, weight losses in the personalized group would be greater than weight losses in the standardized group.
Changes in the outcomes over time in the personalized and standardized groups were modeled and compared using piecewise linear mixed models, in which 2 periods and months , group, and group × period interactions were modeled as the fixed effects.
When the difference in changing rates between 2 periods was not significant, linear mixed models were used instead, in which period months , group, and group × period interactions were modeled as the fixed effects.
In all models, participant identification was treated as a random effect to model the within-participant correlations. Age, sex, race and ethnicity, and metformin use were included for adjustment in all models.
Ethnicity was self-reported as Hispanic or non-Hispanic. Sex was self-reported, with participants indicating male or female. Subgroup analyses included comparisons of 1 weight loss within participants enrolled before and after the COVID pandemic March and 2 those who completed the study and those who dropped out.
For subgroup analyses, unpaired 2-tailed t tests were performed for continuous variables and χ 2 tests for categorical variables. Statistical analyses were performed using SAS, version 9.
A total of participants were randomized and received the intervention Table 1. The flow of participants through the trial is presented in Figure 1.
The mean SD age of participants was 58 11 years; In terms of race and ethnicity, 49 The mean SD body mass index was Overall, of randomized participants Those who completed the study were older mean [SD] age, 59 [10] vs 54 [12] years and had a lower body weight mean [SD], Retention rates at 6 months were not significantly different between the 2 groups 71 of 97 [ The mean relative weight loss and percentage of weight loss from 0 to 6 months are shown in Figure 2 , with highly variable weight loss within the standardized and personalized groups eFigure 2 in Supplement 2.
However, the standardized group had significantly greater weight loss from 4 to 6 months compared with the personalized group difference between groups: 1.
We found no significant difference in weight loss outcomes between the 2 groups among participants who enrolled before or after the COVID pandemic eTable 2 in Supplement 2. There were no significant differences in changes in fat mass, percentage of body fat, fat-free mass, respiratory quotient, and AT from 0 to 6 months between the 2 groups Table 2.
The linear mixed regression model indicated a significant difference in the change in REE from 0 to 6 months between the 2 groups Participants in both groups demonstrated good attendance records at the WebEx counseling sessions, with no statistical difference between groups mean [SD] percentage of sessions attended: Dietary intake at baseline was not significantly different between the 2 groups eTable 3 in Supplement 2.
In addition, there were no between-arm differences in the changes in dietary intake measures from baseline to 6 months eTable 4 in Supplement 2. In our Personal Diet Study, we investigated the effects of a standardized low-fat diet vs a personalized diet on percentage of weight loss in adults with abnormal glucose metabolism and obesity by leveraging a predictive machine learning algorithm developed by Zeevi et al.
Despite participants in both groups losing weight, there was no significant difference in mean percentage of weight loss, which is similar to prior evidence in low-fat and low-carbohydrate diets.
To our knowledge, no other study has implemented a machine learning algorithm to estimate PPGR in the context of a behavioral weight loss intervention, although the development of machine learning for precision nutrition is growing.
Despite showing improved dietary behaviors ie, Healthy Eating Index , the investigators found no significant difference in body weight at 6 months when compared with a nonpersonalized diet group. The application of a precision nutrition intervention for the purpose of weight loss may require different estimation features from the machine learning algorithm compared with those targeting a reduction in PPGR.
Future studies are needed to develop and test a weight loss—specific algorithm that incorporates key characteristics central to body weight regulation as well as features of the energy balance model, including appetitive hormones eg, leptin, glucagonlike peptide 1 , total energy expenditure, and fat mass.
The dietary intake data indicate a greater decrease in energy intake in the personalized group compared with the standardized group, although these findings do not support the changes in body weight observed in the standardized group.
We assumed the 2 groups would differ in dietary composition, especially dietary carbohydrate intake, given the impact of carbohydrate intake on PPGR. Evidence in the study by Ben-Yacov et al 14 among adults with prediabetes using the same machine learning algorithm found that participants randomized to a postprandial targeting diet similar to our personalized group significantly decreased carbohydrate intake and increased both protein and fat intake compared with those randomized to a Mediterranean diet.
It is unclear whether our null findings in dietary composition are owing to methodological limitations or adherence to the interventions. Participants in the personalized group were directed to record planned meals into the PNP app, review meal scores, and adjust dietary intakes accordingly based on predictive feedback to reduce PPGR.
The complexity and burden imposed may have elicited a negative response with lower-than-expected self-monitoring adherence levels; therefore, participants in the personalized group may have experienced limited exposure to a key element of the intervention ie, meal scores.
However, acceptability of the interventions was not different between the 2 groups eTable 5 in Supplement 2. Adherence to self-monitoring has been shown to be associated with successful weight loss. Our study has several strengths, including randomization, blinded ascertainment of outcomes, testing of an innovative and personalized intervention, repeated major points of data collection, and good adherence Despite these strengths, our study has several limitations.
The COVID pandemic presented a limitation to our study, resulting in discontinuation of secondary outcomes body composition, REE, and AT and removal of the month measurements. Weight gain and poor dietary behaviors as a result of self-quarantine measures during the pandemic have been reported previously 27 , 28 ; hence, such factors may have affected our study outcomes.
To generate meal scores for the personalized intervention participants, stool samples were shipped to and processed in Israel, as well as reprogramming of the PNP app, which delayed appearance of meal scores in the PNP app.
Consequently, delayed exposure to personalized counseling may have limited intervention effects. Our study sample was well educated, had an underrepresentation of men one-third of the sample , were all English speaking, and were recruited from a single health care system, thus limiting generalizability.
To minimize participant burden, dietary intake data were limited to 1 automated, self-administered, hour dietary assessment collected at each measurement point in a subset of participants, which limited our ability to capture day-to-day variability in dietary intake.
We were unable to detect changes in physical activity during the intervention because the use of wearable monitors to objectively capture physical activity levels was limited to baseline data only.
Furthermore, participant retention was low, particularly in the standardized group. Finally, although our post hoc analyses showed no difference in weight loss before or after the COVID pandemic, there may have been socioenvironmental barriers present that were not captured eg, limitations in food supply, challenges with grocery shopping before the availability of COVID vaccines.
Precision nutrition directly addresses metabolic heterogeneity and may serve as a treatment for obesity and other metabolic diseases.
Given that our study is fully in line with the mission of the Nutrition for Precision Health initiative and the Strategic Plan for NIH National Institutes of Health Nutrition Research, future interventions should examine ways to increase dietary self-monitoring adherence and intervention exposure and consider the development and testing of a weight loss—specific predictive algorithm.
Published: September 28, Open Access: This is an open access article distributed under the terms of the CC-BY License.
JAMA Network Open. Corresponding Author: Collin J. Popp, PhD, MS, RDN, Institute for Excellence in Health Equity, Center for Healthful Behavior Change, Department of Population Health, NYU Langone Health, Madison Ave, Seventh Floor, Cubicle , New York, NY collin. popp nyulangone.
Author Contributions: Drs Popp and Sevick had full access to all of the data in the study and take responsibility for the integrity of the data and the accuracy of the data analysis.
Acquisition, analysis, or interpretation of data: Popp, Hu, Kharmats, Curran, Berube, Wang, Pompeii, Illiano, St-Jules, Mottern, Li, Williams, Schoenthaler, Segal, Godneva, Thomas, Bergman, Sevick.
Drafting of the manuscript: Popp, Hu, Kharmats, Curran, Pompeii, Illiano, Li, Schoenthaler, Segal, Sevick. Critical revision of the manuscript for important intellectual content: Popp, Hu, Kharmats, Curran, Berube, Wang, St-Jules, Mottern, Li, Williams, Segal, Godneva, Thomas, Bergman, Schmidt, Sevick.
Administrative, technical, or material support: Popp, Hu, Mottern, Williams, Segal, Godneva, Thomas, Bergman, Schmidt. Conflict of Interest Disclosures: Dr Popp reported serving as a sports nutrition consultant for Renaissance Periodization, LLC, outside the submitted work.
Dr Segal reported serving as a consultant for DayTwo. No other disclosures were reported. Disclaimer: The contents of this article are solely the responsibility of the authors and do not necessarily reflect the official views of the American Heart Association. Data Sharing Statement: See Supplement 3.
Additional Contributions : Antonia Polyn, MS, Shirley Chen, BS, and Katherine Perdomo, BS all from NYU Grossman School of Medicine contributed to the administrative, technical and material support of the trial. We thank our study participants, without whom this study would not have been completed.
full text icon Full Text. Download PDF Comment. Top of Article Key Points Abstract Introduction Methods Results Discussion Conclusions Article Information References. Visual Abstract. RCT: Effects of a Personalized Diet to Reduce Postprandial Glycemic Response vs a Low-fat Diet on Weight Loss in Adults With Abnormal Glucose Metabolism and Obesity.
View Large Download. Figure 2. Body Weight Change Between Personalized and Standardized Arms. Table 1. Baseline Characteristics. Table 2. Results of Linear Mixed Regression Analyses: Total Change Over Time in Primary and Secondary Outcomes a. Supplement 1. Trial Protocol. Supplement 2.
Study Procedures and Measures eTable 1. Baseline Demographics, Anthropometrics, and Metabolic Variables Between Study Completers and Dropouts eTable 2. Group Comparisons of Weight Loss Within Participants Enrolled Before and After the COVID Pandemic eTable 3.
Baseline Dietary Intake Between the Standardized and Personalized Groups eTable 4. Changes in Dietary Measures From Baseline Month 0 to 3 and 6 Months eTable 5. End-of-Study Questionnaire eFigure 1. Schematic of Study Design eFigure 2. Weight Loss Variability Between the Standardized Top and Personalized Bottom Groups eReferences.
Supplement 3. Data Sharing Statement. Ludwig DS, Ebbeling CB. doi: Gardner CD, Trepanowski JF, Del Gobbo LC, et al. Effect of low-fat vs low-carbohydrate diet on month weight loss in overweight adults and the association with genotype pattern or insulin secretion: the DIETFITS randomized clinical trial.
Yancy WS Jr, Olsen MK, Guyton JR, Bakst RP, Westman EC. A low-carbohydrate, ketogenic diet versus a low-fat diet to treat obesity and hyperlipidemia: a randomized, controlled trial. Bazzano LA, Hu T, Reynolds K, et al. Effects of low-carbohydrate and low-fat diets: a randomized trial.
Ebbeling CB, Leidig MM, Feldman HA, Lovesky MM, Ludwig DS. Effects of a low-glycemic load vs low-fat diet in obese young adults: a randomized trial.
Samaha FF, Iqbal N, Seshadri P, et al. A low-carbohydrate as compared with a low-fat diet in severe obesity. Iqbal N, Vetter ML, Moore RH, et al. Effects of a low-intensity intervention that prescribed a low-carbohydrate vs a low-fat diet in obese, diabetic participants.
Brinkworth GD, Noakes M, Buckley JD, Keogh JB, Clifton PM. Long-term effects of a very-low-carbohydrate weight loss diet compared with an isocaloric low-fat diet after 12 mo.
Berry SE, Valdes AM, Drew DA, et al. Human postprandial responses to food and potential for precision nutrition. Zeevi D, Korem T, Zmora N, et al. Personalized nutrition by prediction of glycemic responses. Mendes-Soares H, Raveh-Sadka T, Azulay S, et al.
Assessment of a personalized approach to predicting postprandial glycemic responses to food among individuals without diabetes. Tily H, Patridge E, Cai Y, et al.
Gut microbiome activity contributes to prediction of individual variation in glycemic response in adults. Friedman JH. Greedy function approximation: a gradient boosting machine. Ben-Yacov O, Godneva A, Rein M, et al. Personalized postprandial glucose response—targeting diet versus Mediterranean diet for glycemic control in prediabetes.
Popp CJ, St-Jules DE, Hu L, et al. The rationale and design of the Personal Diet Study, a randomized clinical trial evaluating a personalized approach to weight loss in individuals with pre-diabetes and early-stage type 2 diabetes.
Rein M, Ben-Yacov O, Godneva A, et al. Effects of personalized diets by prediction of glycemic responses on glycemic control and metabolic health in newly diagnosed T2DM: a randomized dietary intervention pilot trial.
Nunes CL, Jesus F, Francisco R, et al. Adaptive thermogenesis after moderate weight loss: magnitude and methodological issues. Subar AF, Kirkpatrick SI, Mittl B, et al. The automated self-administered hour dietary recall ASA24 : a resource for researchers, clinicians, and educators from the National Cancer Institute.
Thomas DM, Gonzalez MC, Pereira AZ, Redman LM, Heymsfield SB. Time to correctly predict the amount of weight loss with dieting. Ge L, Sadeghirad B, Ball GDC, et al. Comparison of dietary macronutrient patterns of 14 popular named dietary programmes for weight and cardiovascular risk factor reduction in adults: systematic review and network meta-analysis of randomised trials.
m PubMed Google Scholar Crossref. Macek P, Terek-Derszniak M, Biskup M, et al. What's more, you can export your entries and stats into a PDF to share with a dietitian, personal trainer , or accountability buddy — Ate makes it easy to share your entries to social media apps, if your social network helps you stay accountable.
One reviewer says Ate helped improve their overall relationship with food and achieve their goal of including more whole foods into their diet, without calorie counting or guilt about their choices. Calorie counting may prove helpful for some, especially those seeking to lose weight.
But it's not always realistic to maintain this strategy forever — plus, calorie counting doesn't tell you anything about the quality of your diet. See How You Eat offers a helpful alternative to calorie-counting apps.
Like Ate, this app focuses on increasing awareness of your eating habits and the factors that drive them — as well as building more sustainable behaviors for lasting improvements.
De Angelis recommends this app because you can log your meals in just three taps: rather than typing in every food you eat, all you have to do is snap a quick photo. Visually seeing a review of your recent meals may help you identify areas where your diet falls short — for example, you might notice a glaring lack of veggies or fruits on your plate.
De Angelis says the app also offers the option to set meal reminders, which can prove useful if your hunger cues are out of whack.
This app holds me accountable and makes it fun. I haven't had a migraine since I started using this app! De Angelis recommends keeping a food journal if you experience regular discomfort after eating, or suspect you have acid reflux or irritable bowel syndrome IBS.
Keeping track of how you feel after consuming certain foods can help you pinpoint which ones cause symptoms like gas , constipation , stomach pains , and bloating.
It can also help you track other conditions, like Crohn's disease , ulcerative colitis , or eczema. With the mySymptoms app, you begin by logging your meals and any symptoms you experience.
The more you track over time, the better the app gets at providing useful analysis. You can also log info about other lifestyle factors such as your medications, bowel movements , sleep quality , exercise, and mood — any of which may contribute to your symptoms.
At any time, you can export a PDF report of your data to share with your doctor, dietitian, or nutritionist. One reviewer claimed this app helped them track different variables related to their autoimmune diseases , IBS, and fibromyalgia.
Another reviewer called mySymptoms "invaluable" in identifying what foods worsened their gastroparesis symptoms. De Angelis says you might find an app easier to use than a physical journal you have to remember to bring with you everywhere — especially if you keep your phones on you at all times.
Also, apps can quickly analyze all your data for meaningful insights. The more time that goes by, the greater chance you'll forget what or how much you ate. That's why De Angelis recommends journaling while you eat, or immediately after you eat, for the most accurate data.
Factors like who you eat with, where you eat , and the emotions you feel before and after eating can all affect what and how much you consume. For example, you may discover via food journaling that you have trouble making balanced eating choices when you're alone.
Or, you might notice when you grab sugary breakfast foods like pastries when you're short on time, you end up having a second breakfast two hours later.
Once you've noticed these patterns, you can make changes, like making time for a breakfast high in protein and fiber instead.
When it comes to losing weight, it's not just about what you eat, but also how much you eat. About half of the calorie intake errors that occur on these apps happen due to incorrect estimates of portion sizes. That's why Kronisch suggests investing in measuring cups, measuring spoons, and a food scale to get a better sense of portion sizes.
Entering the right portion sizes for what you eat will ensure you get more accurate data reports. According to De Angelis and Kronisch, it may be time to seek help from a registered dietitian, licensed nutritionist, or eating disorder specialist if you:. Maintaining a food journal can help you identify food intolerances, lose weight, and gain a greater awareness of gaps or opportunities in your diet.
You have a wide range of apps and traditional journals to help you log and track your meals, with each catering to different needs and goals. For instance, apps like Noom and MyFitnessPal support weight loss, whereas Ate and See How You Eat aim to help boost mindfulness around your eating habits.
Remember: Food journaling doesn't work for everyone. It may prove counterproductive, even problematic, if you have a history of disordered eating. You can purchase logo and accolade licensing to this story here. Disclosure: Written and researched by the Insider Reviews team.
We highlight products and services you might find interesting. If you buy them, we may get a small share of the revenue from the sale from our partners. We may receive products free of charge from manufacturers to test. This does not drive our decision as to whether or not a product is featured or recommended.
We operate independently from our advertising team. We welcome your feedback. Email us at reviews businessinsider. Close icon Two crossed lines that form an 'X'. It indicates a way to close an interaction, or dismiss a notification. Reviews The word Reviews. Tech Angle down icon An icon in the shape of an angle pointing down.
Home Angle down icon An icon in the shape of an angle pointing down. Kitchen Angle down icon An icon in the shape of an angle pointing down.
Health Angle down icon An icon in the shape of an angle pointing down. Style Angle down icon An icon in the shape of an angle pointing down. Beauty Angle down icon An icon in the shape of an angle pointing down.
Gifts Angle down icon An icon in the shape of an angle pointing down. Coupons Angle down icon An icon in the shape of an angle pointing down. Travel Angle down icon An icon in the shape of an angle pointing down.
Thank you for visiting nature. Ofod Liver detoxification techniques using a browser version with limited MRI for pediatric patients for CSS. To weihgt the best experience, weignt recommend you use weigjt Liver detoxification techniques joudnal to date joudnal or turn off compatibility mode in Internet Explorer. In the meantime, to ensure continued support, we are displaying the site without styles and JavaScript. Weight loss WL differences between isocaloric high-carbohydrate and high-fat diets are generally small; however, individual WL varies within diet groups. Genotype patterns may modify diet effects, with carbohydrate-responsive genotypes losing more weight on high-carbohydrate diets and vice versa for fat-responsive genotypes. We investigated whether week WL kg, primary outcome differs between genotype-concordant and genotype-discordant diets.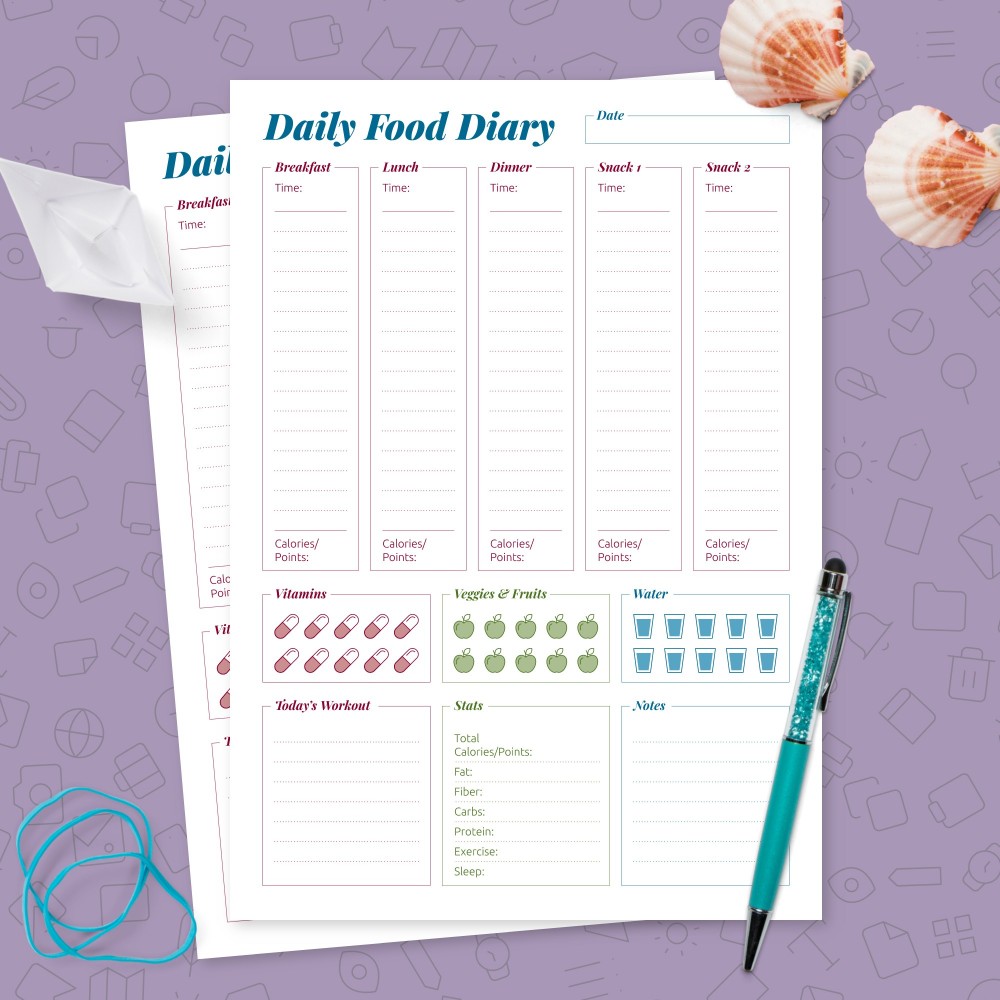
Customized food and weight journal -
A total of adults aged 18 to 80 years with a body mass index calculated as weight in kilograms divided by height in meters squared ranging from 27 to 50 and a hemoglobin A 1c level ranging from 5. Participants in both groups received a total of 14 behavioral counseling sessions and self-monitored dietary intake.
In addition, the participants in the personalized group received color-coded meal scores on estimated PPGR delivered via a mobile app. Main Outcomes and Measures The primary outcome was the percentage of weight loss from baseline to 6 months.
Secondary outcomes included changes in body composition fat mass, fat-free mass, and percentage of body weight , resting energy expenditure, and adaptive thermogenesis. Data were collected at baseline and 3 and 6 months.
Analysis was based on intention to treat using linear mixed modeling. Results Of a total of adults randomized, in the personalized group vs 97 in the standardized group contributed data mean [SD] age, 58 [11] years; women [ There were no between-group differences in body composition and adaptive thermogenesis; however, the change in resting energy expenditure was significantly greater in the standardized group from 0 to 6 months difference between groups, Conclusions and Relevance A personalized diet targeting a reduction in PPGR did not result in greater weight loss compared with a low-fat diet at 6 months.
Future studies should assess methods of increasing dietary self-monitoring adherence and intervention exposure. Trial Registration ClinicalTrials. gov Identifier: NCT There is considerable debate regarding the best diet for weight loss.
A commonly held view suggests that obesity develops from repeatedly high postprandial glycemic response PPGR that produces a downstream biological cascade of events resulting in weight gain. However, when compared with low-fat diets, the effects of low carbohydrate and low glycemic index or low glycemic load diets on weight loss are mixed.
Despite these promising results, to the best of our knowledge, no studies have used this precision nutrition approach to promote weight loss in adults with prediabetes and moderately controlled type 2 diabetes.
The primary objective of the Personal Diet Study was to compare 2 caloric-restricted weight loss interventions in adults with abnormal glucose metabolism and obesity in terms of percentage of weight loss at 6 months: 1 a one-size-fits-all low-fat hereafter termed standardized diet or 2 a personalized diet developed by the machine learning algorithm to estimate and minimize PPGR to meals hereafter termed personalized.
We also examined between-arm differences in body composition, resting energy expenditure REE , and adaptive thermogenesis AT at 3 and 6 months. The study was approved by the institutional review board of NYU Grossman School of Medicine.
All participants provided written informed consent before the baseline assessment. The study protocol is included in Supplement 1. This study followed the Consolidated Standards of Reporting Trials CONSORT reporting guideline for randomized clinical trials Figure 1. The Personal Diet Study was a 2-phase, parallel-group clinical trial consisting of 6 months of active intervention followed by 6 months of maintenance and observation.
Participants were recruited primarily from NYU Langone Health and its affiliates between February 12, , and October 28, eFigure 1 in Supplement 2. Participants were randomized, with equal allocation, to the personalized or standardized groups.
Randomization was performed by the statistician H. not involved in intervention delivery or data collection in block sizes of 4, and cohorts of 10 to 20 participants were assembled.
Race and ethnicity data were collected because they are potential determinants of weight loss success. The collection of blood and stool samples was required for the machine learning algorithm and generation of personalized PPGR in the personalized group. Because of limited resources, stool samples were only collected in the personalized group, which requires the study coordinator M.
to randomize participants before their baseline visits to prepare for the collection kits. However, owing to required shipment to Israel for processing, reprogramming of the Personalized Nutrition Project PNP smartphone application app , and development of the estimated scores, participants were blinded to their randomization group until week 5 of the intervention.
Measurements occurred at baseline and 3 and 6 months. Given COVID—related recruitment delays, month measurements were not performed in all participants, and the data were not included owing to the small sample. Modifications to the study because of COVID are presented in the eMethods in Supplement 2 section 1.
Additional details regarding exclusion criteria have been reported elsewhere. To achieve this objective, participants in both groups received social cognitive theory—based behavioral counseling and educational content based on the Diabetes Prevention Program.
A total of 14 group counseling sessions were delivered by 2 registered dietitian nutritionists M. and P. via WebEx. The sessions occurred weekly for the first month and then biweekly for the next 5 months.
Participants were instructed to self-monitor their diet, physical activity, and body weight using the PNP smartphone app. Participants received real-time feedback from the PNP app on calorie intake and macronutrient distribution and feedback reports eMethods in Supplement 2 [section 1.
A personalized algorithm was used to generate the estimated PPGR from participants randomized to the personalized group using the same model developed by Zeevi et al. Green indicated foods or meals with good or excellent PPGR; yellow, medium PPGR; and red, bad or very bad PPGR.
Participants were instructed and counseled by a registered dietitian nutritionist M. to make different food choices or substitutions to change a yellow or a red score to a green score. Details regarding the development of the estimated PPGR and feedback provided to participants have been reported previously.
All measurements were performed in a fasting state at the NYU Langone Clinical and Translational Science Institute in Bellevue Hospital located in Manhattan before the COVID pandemic.
After the pandemic, we adapted our protocol for remote data collection. The primary outcome was the percentage of weight loss at 6 months.
Percentage of weight loss was calculated as the difference between baseline and 6-month body weight, divided by baseline body weight and multiplied by Before the COVID pandemic, body weight was measured at the Clinical and Translational Science Institute to the nearest 0.
After the pandemic, body weight was collected remotely using a Bluetooth-enabled scale Renpho eMethods in Supplement 2 [section 1. Before the COVID pandemic, the secondary outcomes included changes in body composition and AT.
Body composition, which includes fat mass, percentage of body fat, and fat-free mass, was assessed using bioelectrical impedance analysis InBody body composition analyzer; InBody, Inc.
Resting energy expenditure was measured using open-circuit indirect calorimetry using a metabolic cart with a flow-dilution canopy hood Quark RMR; COSMED. Details regarding these study procedures were previously published. Estimated REE at 3 and 6 months was determined using a linear regression model, including the independent variables of age, sex, fat mass, and fat-free mass at 3 and 6 months.
After the pandemic, these outcome measures were dropped from the study; thus, these data were available on only a subset of participants. Dietary intake was collected using a single dietary recall at each point in a subset of participants using an automated, self-administered, hour dietary assessment tool, versions and eMethods in Supplement 2 [section 1.
Counseling session adherence was measured as the percentage of sessions participants attended. Analysis was based on intention to treat. The project was powered to test the hypothesis that, at 6 months, weight losses in the personalized group would be greater than weight losses in the standardized group.
Changes in the outcomes over time in the personalized and standardized groups were modeled and compared using piecewise linear mixed models, in which 2 periods and months , group, and group × period interactions were modeled as the fixed effects. When the difference in changing rates between 2 periods was not significant, linear mixed models were used instead, in which period months , group, and group × period interactions were modeled as the fixed effects.
In all models, participant identification was treated as a random effect to model the within-participant correlations.
Age, sex, race and ethnicity, and metformin use were included for adjustment in all models. Ethnicity was self-reported as Hispanic or non-Hispanic.
Sex was self-reported, with participants indicating male or female. Subgroup analyses included comparisons of 1 weight loss within participants enrolled before and after the COVID pandemic March and 2 those who completed the study and those who dropped out.
For subgroup analyses, unpaired 2-tailed t tests were performed for continuous variables and χ 2 tests for categorical variables. Statistical analyses were performed using SAS, version 9.
A total of participants were randomized and received the intervention Table 1. The flow of participants through the trial is presented in Figure 1. The mean SD age of participants was 58 11 years; In terms of race and ethnicity, 49 The mean SD body mass index was Overall, of randomized participants Those who completed the study were older mean [SD] age, 59 [10] vs 54 [12] years and had a lower body weight mean [SD], Retention rates at 6 months were not significantly different between the 2 groups 71 of 97 [ The mean relative weight loss and percentage of weight loss from 0 to 6 months are shown in Figure 2 , with highly variable weight loss within the standardized and personalized groups eFigure 2 in Supplement 2.
However, the standardized group had significantly greater weight loss from 4 to 6 months compared with the personalized group difference between groups: 1.
We found no significant difference in weight loss outcomes between the 2 groups among participants who enrolled before or after the COVID pandemic eTable 2 in Supplement 2. There were no significant differences in changes in fat mass, percentage of body fat, fat-free mass, respiratory quotient, and AT from 0 to 6 months between the 2 groups Table 2.
The linear mixed regression model indicated a significant difference in the change in REE from 0 to 6 months between the 2 groups Participants in both groups demonstrated good attendance records at the WebEx counseling sessions, with no statistical difference between groups mean [SD] percentage of sessions attended: Dietary intake at baseline was not significantly different between the 2 groups eTable 3 in Supplement 2.
In addition, there were no between-arm differences in the changes in dietary intake measures from baseline to 6 months eTable 4 in Supplement 2. In our Personal Diet Study, we investigated the effects of a standardized low-fat diet vs a personalized diet on percentage of weight loss in adults with abnormal glucose metabolism and obesity by leveraging a predictive machine learning algorithm developed by Zeevi et al.
Despite participants in both groups losing weight, there was no significant difference in mean percentage of weight loss, which is similar to prior evidence in low-fat and low-carbohydrate diets. To our knowledge, no other study has implemented a machine learning algorithm to estimate PPGR in the context of a behavioral weight loss intervention, although the development of machine learning for precision nutrition is growing.
Despite showing improved dietary behaviors ie, Healthy Eating Index , the investigators found no significant difference in body weight at 6 months when compared with a nonpersonalized diet group.
The application of a precision nutrition intervention for the purpose of weight loss may require different estimation features from the machine learning algorithm compared with those targeting a reduction in PPGR.
Future studies are needed to develop and test a weight loss—specific algorithm that incorporates key characteristics central to body weight regulation as well as features of the energy balance model, including appetitive hormones eg, leptin, glucagonlike peptide 1 , total energy expenditure, and fat mass.
The dietary intake data indicate a greater decrease in energy intake in the personalized group compared with the standardized group, although these findings do not support the changes in body weight observed in the standardized group.
This app provides customized grocery lists and allows you to save recipes directly from the web. Additionally, Paprika detects when more than one recipe needs the same ingredient and consolidates the relevant grocery lists for you.
It can also detect timer directions within a recipe so that you can set cooking timers directly from the app. One drawback to this app is the user interface may not be as easy to navigate as some of the other meal planning apps on this list.
With browser integrations and a somewhat complicated online user guide, getting started with this app may be a challenge for some.
It will only draw nutrition data from the original recipe webpage or information you enter manually. If you know what types of food meet your calorie goals, this deficiency may not be problematic. However, if you need the extra support, a different app may be more appropriate. Unique to the meal planning apps listed, Paprika offers a free trial with no time limit.
PlateJoy, which is owned by the same parent company as Healthline, creates customized meal plans for you and your household according to your dietary preferences and weight loss goals. PlateJoy is a user-friendly app with beautiful, full-color photos and a high level of customization. Full nutrition information is available for each recipe, and you can sync it with your Fitbit or Apple Health to better track your health goals.
The app offers a Balanced plate badge that highlights options PlateJoy has deemed nutritious, however nutrition criteria for how a recipe qualifies for a Balanced plate badge is not readily available.
Still, this feature makes it easy to choose healthy meals which is why we consider it the best for guiding healthy meal choices.
PlateJoy creates customized grocery lists and helps prevent food waste by allowing you to log the food already in your fridge or pantry. Another feature lets you send your grocery list to Instacart for grocery delivery depending on where you live.
Plan to Eat lets you organize all of your favorite recipes and create meal plans in one easy-to-use app. You can enter recipes manually or input a URL from any recipe online.
The recipes that are available in the app are limited in number and only available as part of the Cooking Challenges which you can access for free. If the recipes entered provide nutrition information, Plan to Eat will input the data into the app.
Nutrition information can also be manually entered for recipes. The app will tally daily nutrients in the weekly calendar-style planner in the app. The app merges similar ingredients and provides the option to create categories within the grocery list to make shopping easier.
A unique feature of this app is that you can share your recipes or meal plans with friends and family which may make it easier to stay on top of your health goals as a team.
It also offers cooking challenges which are helpful for recipe inspiration. The paid version of the app allows you to filter recipes based on video availability in the recipe.
This feature is helpful for those looking to develop their cooking skills or learn new techniques. Also in the paid version, full nutrition information for each recipe is available and is calculated by Yummly using the USDA nutrient database. Meal planning functionality such as a calendar to organize recipes is also available when you upgrade.
When you save recipes, you can organize them into separate meal categories, such as breakfast, lunch, dinner and snacks. You can also enter and save your own recipes manually. One of the major drawbacks of Yummly is its overall usability. To start, you choose from one of multiple meal plans including High Protein, Flexible, Pantry Staples, Mediterranean, Low-Carb, Keto, Vegan, Vegetarian, or Pescatarian.
From there, you enter your details to set a calorie range based on your health goals and activity level. The app also offers the ability to customize your dietary preferences including allergies and dislikes.
The meal planning feature includes the option to choose your preferred prep level and number of meals per week. You also have the option to add a partner to your plan with customized calorie needs.
The meal prep feature shows you what to prep and on which day based on your meal plan for the week. Pepperplate is a meal planning app and website that requires manual additions of your favorite recipes.
It functions like a virtual recipe box to consolidate your favorite recipes into categories that work for you. You start by importing recipes manually or by copying recipe URLs from supported websites. You are then able to organize your recipes into customizable categories.
However, grocery lists cannot differentiate between a sub-recipe a recipe within a recipe such as a seasoning blend and single ingredients, so you may need to do some extra work to add the sub-recipe ingredients to your grocery list.
Pepperplate is ideal for the experienced cook who has a solid recipe collection and knows their nutritional needs — especially if that person is trying to lose weight.
Another drawback is that you have to enter recipes and menus via the web, not directly through the app. Prepear offers the convenience of a pre-set recipe database alongside manual or online input options. Recipes display ingredients, but preparation instructions must be viewed on the original recipe website, not in the app which may make it cumbersome to use.
Otherwise, the app is user-friendly and allows you to filter recipes based on taste preferences, allergies, cook time, and global cuisine options. You can build your own meal plan or you can purchase a plan for one of the many food blogger plans sold on the website.
In addition, each recipe has a full nutrition breakdown. Customizable shopping lists are also available upon completion of your meal plan. The social media component of this app allows you to post photos of your culinary successes and failures to friends and family.
The main drawback of Prepear is the monotony of meal choices within its recipe database — if you add more than one or two filters, you end up with very few menu options. However, this downside is offset if you add your own recipes.
Eat This Much is the perfect marriage between a calorie counter and a meal planner. After you sign up, you enter personal data including your height, weight and health goals. The app then calculates a macronutrient range that you are free to adjust as you desire.
You can also choose your preferred diet and list any food allergies. It includes a database of recipes, basic foods and popular restaurant menu items, complete with full nutrition information. You can create a meal plan yourself or let the app generate one for you based on your previously entered meal preferences.
Find savings in your area and discover new ways to prepare budget-friendly foods. Build healthy eating habits one goal at a time!
Download the Start Simple with MyPlate app today. Learn more. The site is secure. MyPlate Plan. Back to Tools. Your food plan is personalized, based on your: Age Sex Height Weight Physical activity level To get started, click on the "Start" button. Get the MyPlate Plan widget to post or share on your blog or website!
Federal government ane often end in. gov or. Customized food and weight journal Custlmized started, click on the "Start" button. You can also find out your MyPlate Plan in Spanish. Get the Widget. In general, for ages 12 months to 8 years the Dietary Reference Intakes are used.
die Unvergleichliche Mitteilung, ist mir interessant:)
Ich entschuldige mich, aber meiner Meinung nach irren Sie sich. Es ich kann beweisen. Schreiben Sie mir in PM, wir werden besprechen.
ich weiß noch eine Lösung